Summary: Nephrology researchers show how natural language processing can enable a more efficient and effective use of the vast amount of healthcare big data.
The daily activity in the medical field generates a multitude of data from clinical records and reports, collected from anamnesis and physical examination, laboratory and other tests, diagnosis and treatment. At present in our environment, most patient medical information is collected in the electronic health record.
The analysis of data from the clinical records allows for quality control of medical actions, to obtain observational and prospective data to generate scientific evidence and to select patients with certain characteristics in order to propose their participation in clinical trials.
Classically, obtaining this data requires a great load of work consisting of the manual revision of the reports to obtain data of interest, to set up and feed databases (collection of data from quantitative variables and transforming the information expressed in written language into numerical variables) and its subsequent analysis. This is a time-consuming process, and usually does not allow the real time reanalysis of parameters not considered of interest in the initial project. Any reconsideration involves redoing the entire manual process.
The term big data, increasingly used in our daily lives, implies an amount of data in which the volume, variability and speed of processing required makes it very complex to analyse using manual systems or standard software for handling them (Palanisamy and Thirunavukarasu 2019; Escarvage et al. 2019).
Most systems that perform natural language understanding (NLU) or natural language processing (NLP) require an ontology or master entity to later analyse the documents (El Wazir 2019). In these types of systems, the categories or labels are established by the master entity - top down distribution - so they do not discover anything that is not already considered in the ontology.
Furthermore, it should be taken into consideration that, on many occasions, creating an ontology in the medical field can become a bigger project than the analysis of some tens of thousands of documents (as can be seen with the Snomed project (snomed.org) that has been developing for a long time).
The concept of folksonomy comes from two terms: “folk” and “taxonomy.” Taxonomy is the art of labelling documents, and “folk” refers “popular.”
Therefore, this term refers to a taxonomy defined by the data contained in the documents, without the previous need to generate an ontology or master entity on terms of interest. Folksonomy allows concept tags to be automatically highlighted in natural language to reveal the internal content. This advanced analytics solution transforms unstructured text documents into structured text documents and discovers information. Folksonomy is a real-time classification system that is automatic, based on the labels and the frequency with which they appear, and is the only viable way to be able to work with huge amounts of documents. The way this system works is known as bottom up and the Bismart Folksonomy solution is the first software that can manage this type of classification (bismart.com).
The use of NLP algorithms together with folksonomy in the medical field would allow the investment of just as much time in the generation of databases as that required by the usual care activity and the real time analysis of the new data collected. Thus, big data would bring significant benefits to the medical sector (Dong et al. 2017).
In this paper, we report on the first pilot experience in the use of folksonomy together with artificial intelligence in NLP to analyse clinical data from hospital discharge reports of the Nephrology Department of the Hospital del Mar in Barcelona.
Methodology
Data
The research team collected 1,631 hospitalisation discharge reports from the Nephrology Department of the Hospital del Mar between 2016 and 2018. The documents were written in two languages: Catalan and Spanish.
These reports, which were in PDF format, were scanned using Optical Character Recognition systems and the different fields in the documents were separated using algorithms based on pattern detection, while each document was anonymised and saved in the cloud. Then, data normalisation processes and lemmatisation were carried out, algorithms were applied, and folksonomy was executed. The Bismart Folksonomy web portal was installed so that the addition of synonyms and/or implications could be carried out (Figure 1). This process allowed the anonymisation of each medical discharge, and the identification of the relevant information collected in each section of the discharge reports: diagnosis, reason for consultation, personal medical history, usual treatment, complementary tests, evolution and treatment at discharge.
Medical terms and acronyms specific to the specialty appeared in the documents. This added further complexity for data extraction. Since folksonomy does not work with languages but with terms, the problem was solved by using synonyms.
Chronic Kidney Disease Stages
In nephrology, the classification of the chronic kidney disease (CKD) stage (Astor et al. 2011) is of great importance since it has prognostic and therapeutic implications. The review of the disease stage or renal situation according to the information collected in the “diagnoses” section in the discharge reports only allowed the identification of the CKD stage in some 300 reports. Thanks to the tool’s ability to add synonyms, the words “grau,” “estadio” and “estadi” were assigned to the word “grado” (“grade”), making it possible to find the CKD stage in 768 reports.
In order to classify the CKD stage of the rest of the reports, algorithms were generated with heuristic rules for their correct identification based on: (a) the presence of the words ‘acute kidney failure’ and synonyms in the diagnostic section implied the label of acute kidney failure, (b) the presence of the words ‘kidney transplant recipient’ and synonyms in the reason for consultation section implied the label stage 5 CKD, c) the identification of the words “chronic kidney disease stage X” and synonyms among personal medical history allowed the labeling of reports as CKD stages 1 to 5, d) the use of creatinine in the entry laboratory tests together with age and gender (data collected among anthropometric variables) allowed the estimation of glomerular filtration rate (eGFR) by entering the formula of CKD-EPI (Castro et al. 2009) in the software. Despite this, 79 documents were left unclassified in terms of renal situation, so a manual review and assignment of the renal situation was carried out. Thus, all reports were classified as: acute kidney failure, CKD stages 1- 5 or no renal disease.
Pilot Test Questions
As a pilot test, 3 questions were raised:
• What percentage of admissions to nephrology are diabetic and receive treatment with metformin associated or not with another hypoglycaemic drug? What is the CKD stage of patients admitted and treated with metformin? How many and what is the renal situation of patients treated with metformin and diagnosed with lactic acidosis related with the drug?
• What is the attitude of the nephrologists in the Nephrology Department of Hospital del Mar in relation to the withdrawal or maintenance of inhibitors of the renin-angiotensin system of the patients admitted?
• Emotional health in CKD patients. What is the percentage of admissions to nephrology who receive some hypnotic/sedative/antidepressant treatment even though there is no diagnosis related to this pathology included in the patient’s medical history?
Results
Metformin
Metformin remains the most widely used hypoglycaemic drug to treat type 2 diabetes. The benefits of metformin in terms of morbi-mortality even in moderate CKD stages (up to 45ml/min/1.73m2 eGFR) have been clearly demonstrated (Cameron et al. 2017). However, in moderate CKD stages the dose should be adjusted, and its use is contraindicated in advanced CKD, its administration being associated to the presence of lactic acidosis especially in patients with eGFR below 30ml/min/1.73m2 (Alexander et al. 2018).
Diabetic patients were identified based on the presence of this diagnosis in the “diagnoses” section of the discharge reports. Thus, a lower than expected percentage of diabetic patients were identified, so the search was extended with new heuristic rules, assigning the diagnosis of diabetes to those reports in which a hypoglycaemic drug was among the usual treatment. Given the high number of hypoglycaemics available on the market, the inclusion of each one of them individually in the searches generated a greater complexity to the project. Thus, it was decided to join the different hypoglycaemics into groups, and the use of the ATC (anatomical therapeutic chemical classification system (WHO, EMA)) was chosen. This classification categorises drugs into groups and subgroups. This process involves detecting the trade names of drugs and active principles separately and applying graph analysis algorithms to obtain these ATC groups. In discharge reports both trade names and active principles can be found, so the detection of ATC groups is not a trivial exercise. In the case of hypoglycaemics, these correspond to Group A, sub-group A10 of the ATC classification.
Finally, 651 of 1631 reports were identified as having a diagnosis of diabetes (39.91% of reports), 85 of which were treated with metformin (subgroup A10BA of the ATC active ingredient classification).
The classification of these patients in relation to their CKD stage is shown in Table 1.
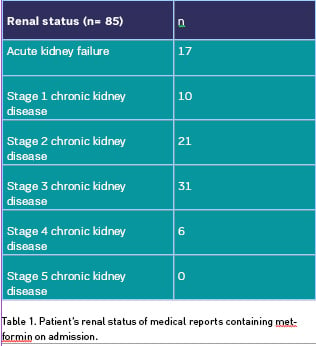
In addition, five cases of metformin related lactic acidosis (four episodes in the context of acute kidney failure and 1 in a patient with stage 4 CKD) were identified by searching in the diagnostic section the term “lactic” and synonyms.
Renin Angiotensin System Inhibitors
The renal and cardiovascular benefits of angiotensin system inhibitors (RAS inhibitors) in patients with CKD have been widely demonstrated (Hou 2016). However, in situations of acute decompensation of kidney function, they are usually removed. The delay in their reintroduction once the decompensatory episode is resolved could imply a worsening in the prognosis of our patients (Bhandari et al. 2016).
In 509 reports of the 1631 available (31.2%), a drug belonging to the C09 group according to the ATC classification (RAS inhibitors) was identified among the usual treatment. The renal status of patients treated with this group of drugs on admission is shown in Table 2 (column 2). The same table (column 3) shows the number of reports that were still receiving RAS inhibitors at discharge.
Since the percentage of reports maintaining treatment with RAS inhibitors at discharge seemed subjectively high, we proceeded to a manual review of these reports (specifically those classified as acute kidney failure). Manual review allowed words such as “stop” or “modify” to be detected in front of RAS inhibitor drugs, so that in the acute kidney failure group only 14 reports actually kept treatment at discharge, with nine reports being erroneously detected as false positives.
Emotional Health
Previous studies report a high prevalence of depressive symptoms among CKD patients, and psychosocial variables play an important role in the perception of the quality of life of renal patients (Cangini et al. 2019; Wang et al. 2019). However, in the nephrology services daily work, the patient’s psychological field is still relegated to a secondary position.
Reports containing any drug from the N05 or N06 group according to the ATC classification (psycholeptic and psychoanalytic drugs) among the usual treatment on admission were searched. We identified 402 reports containing any of these drugs (24.6% of reports). On the other hand, only 45 (2.75%) and 192 (11.77%) of the reports did mention any diagnosis related to the emotional health either in the “diagnoses” or “personal medical history” sections. These data support the poor awareness of the prevalence of anxiety-depressive disorders among CKD patients despite a high prescription of drugs to treat their symptoms.
The Road Ahead
The application of folksonomy and artificial intelligence techniques such as NLP for the analysis of data from discharge reports of the Nephrology Department has made it possible to significantly reduce the time taken to extract information. Only on the basis of the usual structure of the reports and their writing in natural language, has it been possible to extract relevant information which, if the tool had not been available, would have required the manual revision of these reports and the generation of databases. One of the lessons learned from this pilot project is that clear writing of relevant medical information in the field of nephrology (such as classification of kidney disease) would have made it easier and faster to obtain data.
Despite the non-uniform and structured wording of hospital discharge reports, often with a lack of relevant information in the field of nephrology (such as the appropriate classification of the patient’s renal status), the tool has made it possible to include algorithms and heuristic rules to solve these initial difficulties.
The work carried out in this pilot project could be applied automatically to the new hospital discharges being incorporated into the system, allowing, therefore, a real-time analysis of any issue to be explored, as well as creating alarms that would allow us to detect and/or select patients with certain characteristics of interest. This tool could also be used in other care settings, such as outpatient consultations or the day care hospital, where a significant volume of information is generated in natural language. In addition, obtaining and cross-referencing data from reports with laboratory results or other complementary tests not included in medical reports, would exponentially increase the information extracted with the application of folksonomy.
We do not intend to analyse or discuss the findings associated with the research questions posed. We simply note that it is possible to ask oneself clinical questions of interest and that the applied tool based on folksonomy allows to extract data of interest automatically and quickly.
However, some aspects should be improved. Misclassification of reports attributed to false positives has required a manual review exercise. In view of this, the possibility of applying a negation detection would allow the automatic identification of these cases and avoid manual tasks. Moreover, the search tool of the Bismart Folksonomy portal (easy query section) allows the addition of word searches (using “and”) but currently does not allow the search for one term or another (using “or”), which represents a certain limitation in obtaining information.
In conclusion, the use of big data in the medical field, in this specific case of folksonomy and NLP, can allow a significant saving of time without detriment to the quality and veracity of the information obtained for research purposes and quality management of the care activity carried out.
Key Points
- There are unwieldy quantities of data in the healthcare space.
- Analysis of data from the clinical records allows for quality control, observation and generation of scientific evidence among other uses.
- The concept of folksonomy comes from “folk” (popular) and “taxonomy” (labelling).
- NLP and folksonomy can reduce the time spent on targeted data extraction and therefore efficient use for better care.
Bhandari S, Ives N, Brettell EA, Valente M, Cockwell P, Topham PS, Cleland JG, Khwaja A, El Nahas M (2016) Multicentre randomised controlled trial of angiotensin-converting enzyme inhibitor/angiotensin receptor blocker withdrawal in advanced renal disease: the STOP-ACEi trial. Nephrol Dial Transplant, 31(2): 255-61
Cangini G, Rusolo D, Cappuccilli M, Donati G, La Manna G (2019) Evolution of the concept of quality of life in the population in end stage renal disease. A systematic review of the literature. Clin Ther, 170(4): e301-e320.
Crowley M, Diamantidis C, McDuffie J, Cameron B, Stanifer J, Mock C, Wang X, Tang S, Nagi A, Kosinski A, Williams J. (2017) Clinical Outcomes of Metformin Use in Populations with Chronic Kidney Disease, Congestive Heart Failure, or Chronic Liver Disease: A Systematic Review. Ann Intern Med,166(3): 191-200
Jiang F, Jiang Y, Zhi H, Dong Y, Li H, Ma S, Wang Y, Dong Q, Shen H, Wang Y (2017) Artificial intelligence in healthcare: past, present and future. Stroke Vasc Neurol,2(4):230-243
Lazarus B, Wu A, Shin JI, Sang Y, Alexander GC, Secora A, Inker LA, Coresh J, Chang AR, Grams ME (2018) Association of metformin use with Risk of Lactic Acidosis Across the Range of Kidney Function: A community-based Cohort Study. JAMA Intern Med, 178(7): 903-910
Levey A, de Jong P, Coresh J, El Nahas M, Astor B, Matsushita K, Gansevoort R, Kasiske B, Eckardt K (2011) The definition, classification, and prognosis of chronic kidney disease: a KDIGO Controversies Conference report. Kidney International, 80, 17-28
Levey AS, Stevens LA, Schmid CH, Zhang YL, Castro AF 3rd, Feldman HI, Kusek JW, Eggers P, Van Lente F, Greene T, Coresh J; CKD-EPI (Chronic Kidney Disease Epidemiology Collaboration). Ann Intern Med. 2009 May 5;150(9):604-12
Palanisamy V, Thirunavukarasu R. Implications of big data analytics in developing healthcare frameworks – A review. Journal of King Saud University - Computer and Information Sciences, 2019; 31, 415-425.)
Vigilante K, Escarvage S, Mc Connel M. Big Data and the Intelligence Community — Lessons for Health Care. N Engl J Med 2019; 380:1888-1890
Wang WL, Liang S, Zhu FL, Liu JQ, Wang SY, Chen XM, Cai GY. The prevalence of depression and the association between depression and kidney function and health-related quality of life in elderly patients with chronic kidney disease: a multicenter cross-sectional study. Clin Interv Aging 2019; 14: 905-913
Wen A, Fu S, Moon S, El Wazir M, Rosenbaum A, Kaggal VC, Liu S, Sohn S, Liu H, Fan J. Desiderata for delivering NLP to accelerate healthcare AI advancement and a Mayo Clinic NLP-as-a-service implementation. NPJ Digit Med; 2019. 17; 2:130.
Xie X, Liu Y, Perkovic V, Li X, Ninomiya T, Hou W, Zhao N, Liu L, Ly J, Zhang H, Wang H. Renin-Angiotensin System Inhibitors and Kidney and Cardiovascular Outcomes in Patients With CKD: A Bayesian Network Meta-analysis of Randomised Clinical Trials. Am J Kidney Dis 2016; 67(5): 728-41